Section 6.4 Steepest Descent
The algorithm. Let us illustrate first the steepest descend algorithm with linear search in case of quadratic functions: since
\begin{equation*}
f(x) = \frac{1}{2}\boldsymbol{x}^TA\boldsymbol{x} - b^T\boldsymbol{x} +c,
\end{equation*}
then
\begin{equation*}
g_k(\alpha) = \frac{1}{2}(\boldsymbol{x_k-\alpha\nabla_{x_k}f})^T\, A\,(\boldsymbol{x_k-\alpha\nabla_{x_k}f}) - b^T\boldsymbol{x} + c
\end{equation*}
that has a minimum at
\begin{equation*}
\alpha_k = \frac{\nabla_{x_k}f^T\nabla_{x_k}f}{\nabla_{x_k}f^T\,A\,\nabla_{x_k}f}.
\end{equation*}
Hence we get the following algorithm: - select an initial point \(x\text{;}\)
- select a threshold \(\epsilon>0\text{;}\)
- until \(\|\nabla_{x}f\|<\epsilon\text{,}\) set \(x \to x-\displaystyle\frac{\nabla_{x}f^T\nabla_{x}f}{\nabla_{x}f^T\,A\,\nabla_{x}f}\nabla_{x}f.\)
- print \(x\text{.}\)
\begin{equation*}
f(x,y)=5x^2+4xy+y^2-6x-4y+15\text{.}
\end{equation*}
A direct calculation shows that its minimum is attained at
\begin{equation*}
(x,y) = (-1,4),
\end{equation*}
where the function value is 10. In the code we also put two commented lines (line 10 and 11) where we choose \(a\) with, respectively a constant step size and a diminishing size, so that the reader can easily compare the linear search algorithm with the other two. For instance, with the parameters given in the code, the algorithm reaches the requested tolerance in about 10 steps with the linear search, in about 1000 steps with the diminishing size and about 4000 with the constant step.
Same code + plots
Convergence Are these methods going to converge? In some case, at least ignoring the computational errors, the answer is positive: Theorem 6.4.1.
Let \(x_{k+1}=x_k-C_k\nabla_{x_k}f\) and suppose that:- the smallest eigenvalue of the \(C_k\) is not approaching 0;
- the setpsize is chosen according to the line search rule;
Theorem 6.4.2.
Let \(f(x)=\frac{1}{2}x^TAx+b^Tx+c\text{,}\) with \(A\) symmetric and with all positive eigenvalues, and let \(x^*\) be the position of its minimum. Then, for the steepest descent method:- with \(\alpha_k\) given by line search, \(x_k\to x^*\) for all initial points;
- with fixed step size \(\alpha\text{,}\) the same happens provided that \(\alpha<2/\lambda_Q\text{,}\) where \(\lambda_Q\) is the largest eigenvalue of \(Q\text{.}\)
\begin{equation*}
\|x_{k+1}-x^*\|\leq K\|x_k-x^*\|^p
\end{equation*}
The convergence is linear if \(p=1\) and \(K<1\text{:}\) \(\|x_k-x^*\|\simeq a^k\text{,}\) \(a<1\text{.}\) It is sublinear if \(p=1\) and \(K=1\text{:}\) \(\|x_k-x^*\|\simeq 1/k\text{.}\) It is superlinear if \(p>1\text{:}\) \(\|x_k-x^*\|\simeq a^{2^k}\) for some \(a<1\text{.}\) Theorem 6.4.3.
Let \(f(x)=\frac{1}{2}x^TAx+b^Tx+c\text{,}\) with \(A\) symmetric and with all positive eigenvalues, and let \(x^*\) be the position of its minimum. Then the sequence \(x_k\) generated by the steepeset descent method with exact line search converges to \(x^*\) linearly with \(K=\frac{k(A)-1}{k(A)+1}\text{.}\)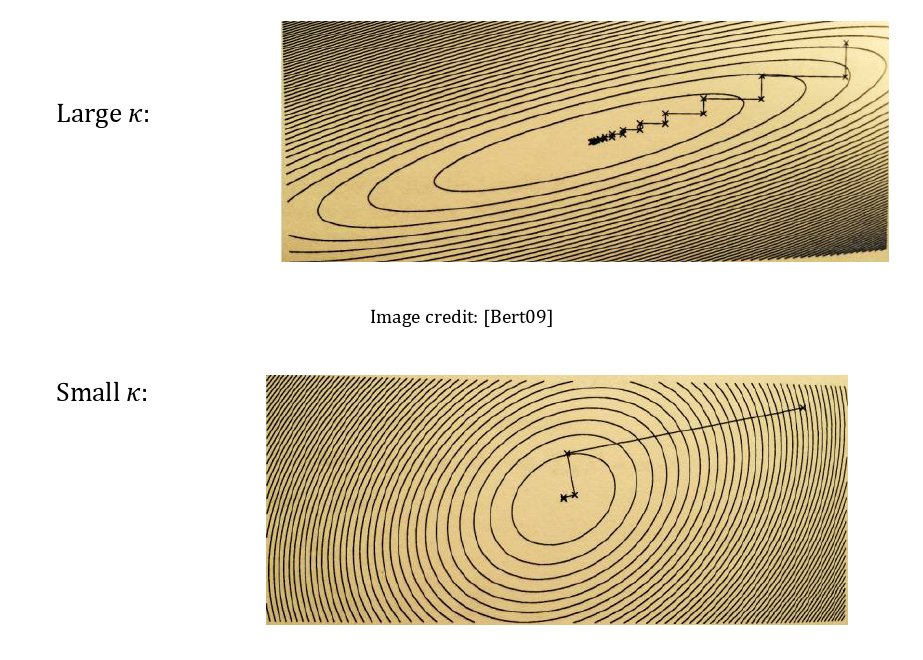